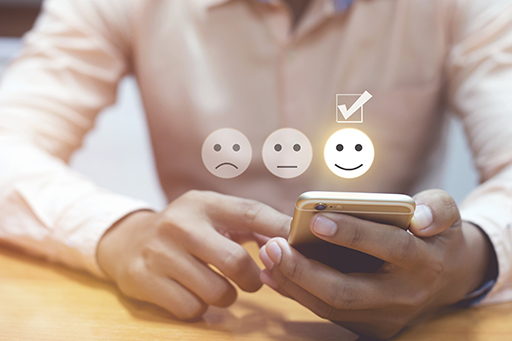
Now more than ever, consumers are talking about brands (and with brands) online. Data about their joy, frustration, and expectations about products and services is an endless source of valuable information for marketing decisions. In this article, we discuss the importance of cultural nuances when training machine learning models for sentiment analysis.
Mentions on social networks, recordings of customers speaking with representatives, emails, reviews, and consumers’ opinions about your products and the competition… The web is flooded with data that can be a competitive advantage for an internationalization process. Sentiment analysis is a valuable technique to help brands understand their markets and improve their marketing strategies and customer experience.
But what happens when a company wants to perform sentiment analysis in different languages? Some of the companies that offer these analytical tools use machine translation to teach their systems how to do sentiment analysis. Automation is key to companies’ operational and economic efficiency; there’s no doubt about it. But when machine translation doesn’t capture cultural nuances and contextual references in comments and reviews, we have to ask ourselves if the data they provide is accurate.
What is sentiment analysis and what is it for
Sentiment analysis is an analytical process that identifies the emotional tone of a text and determines whether it is positive, negative, or neutral. It combines natural language processing and machine learning techniques to assign weighted sentiment scores to words within a sentence or phrase.
Natural Language Processing or NLP is a branch of artificial intelligence that aims to make computers understand how humans speak, write and communicate naturally. NLP allows us to interact with computers as we do with other human beings. A good example of this system in action is customer support chatbots.
For computers to learn from data, Machine Learning (ML) is used. ML is a technique by which computers identify patterns and make decisions. The algorithms are “trained” with data selected and provided by humans specifically for that purpose. The quality of the data used for training directly impacts the quality of the learning process and the results that the model will provide.
Thanks to the speed of information processing provided by machine learning, sentiment analysis has become a very valuable tool for the marketing departments of many companies, providing information to help make decisions and define effective business strategies and objectives.
Here are four ways sentiment analysis can help your marketing strategy:
1. Use customer feedback to improve your service
Finding out what customers like and don’t like about a brand, product, or service is essential for any company. With sentiment analysis, the company can quickly identify and analyze how customers feel about its campaigns and products from the moment they are launched.
2. Research the competition
Businesses can also track mentions of their competitors. Expressions of positive feelings can help you identify where your competitors are succeeding, while negative comments can reveal business opportunities.
3. Prevent and manage crises
Sentiment analysis allows you to follow social mentions in real-time, making it a useful tool for identifying a potential PR crisis that may be developing. Companies can quickly identify a spike in negative sentiment and take immediate action to manage it.
4. Identify influencers
Discovering the influencers talking about your business is another key advantage of sentiment analysis. Some tools score influencer mentions online and allow filtering based on the relevance of the influencer.
The importance of language service providers in multilingual sentiment analysis
Sentiment analysis can be conducted in different languages, depending on the company’s target audience and the markets in which it operates. To obtain quality results, the analysis must be carried out in the language of origin of each market in order to capture the cultural nuances and true meaning of the expressions.
One of the most common and costly mistakes is automatically translating text into a common language like English and then processing the data. Although it may seem like a solution if the data operators communicate in that language, a study reveals that the automatic translation of any textual data can reduce the accuracy of sentiment analysis by up to 20%.
The solution to this problem is to hire a language service provider with experience in big data projects to work alongside the data team on labeling data in their source language. The provider will assemble a team of data annotators who are native speakers of the data’s language and dialect to maximize the quality of the analysis in its original language. It can also help you collect missing data if the machine-learning model requires it.
Choosing the right language service provider for your business can make the difference between buying words as commodities and establishing a strategic alliance that will help your business grow globally. At Go Global, we have been helping large companies maximize the value of their global content for more than a decade. Helping them also take advantage of big data was a natural next step for us. We have the people, the processes, and the infrastructure to do it, but above all, we have the expertise of being a global company helping other global companies to grow in multilingual markets.
Final thoughts
The right multilingual approach to sentiment analysis requires that the machine learning model learns to analyze the opinions and feelings of consumers in the mother tongue in which they are expressed. Only in this way will the system learn to precisely capture the nuances of different languages and linguistic variants.
To ensure quality results, data teams may use a language service provider for data collection and annotation. Having a partner with experience in languages and data management is the safest route to quality data and better business decisions.